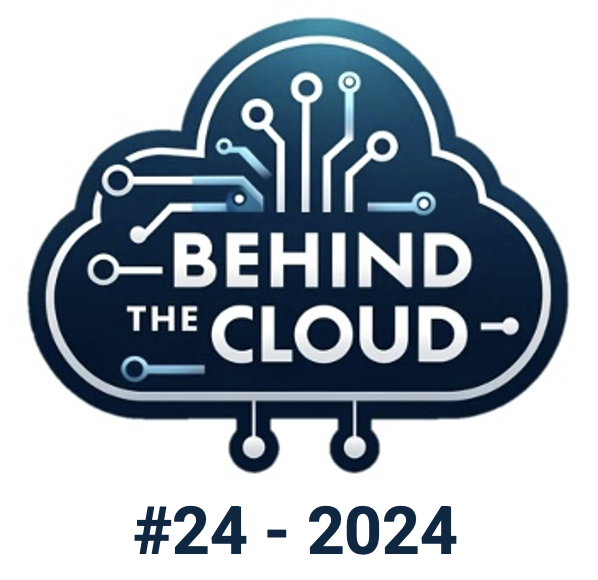
The “Black Box” of AI Investing vs. the Gut Feeling of Fund Managers
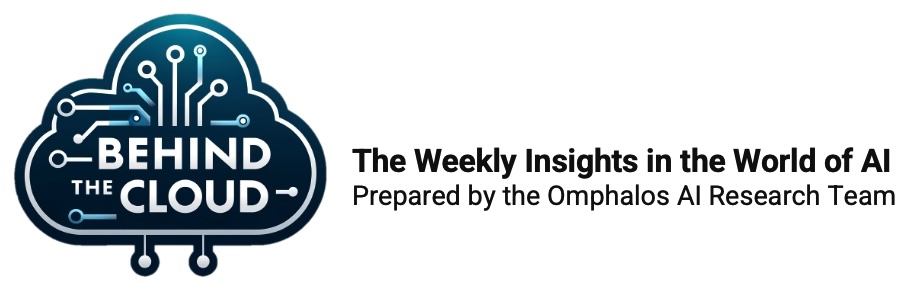
#24 - Behind The Cloud: Demystifying AI in Asset Management: Is It Really a Black Box? (5/6)
The "Black Box" of AI Investing vs. the Gut Feeling of Fund Managers
November 2024
One of the most common concerns surrounding AI-led investing is the perception that it operates as a “black box”—a system that produces outputs (investment decisions) without revealing the logic behind them. This idea of opacity can be unsettling for both asset managers and investors, who are used to more traditional methods of decision-making, such as fundamental analysis or relying on the experience and intuition of human fund managers. But is AI truly a “black box,” and how does it compare to the decision-making process of human fund managers?
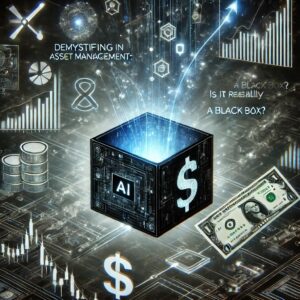
In this chapter, we’ll explore the differences between AI-driven investment strategies and the traditional “gut feeling” approach of active fund managers. We’ll also dive into the factors influencing human decisions and show how AI, while complex, can offer more transparency than it’s often given credit for.
The “Black Box” Perception of AI
The idea that AI operates as a “black box” stems from its complexity and the challenge of understanding how AI models, particularly deep learning and neural networks, make their decisions. These models analyze vast amounts of data, identify patterns, and generate investment signals. However, because the decision-making process involves multiple layers of computations, it can be difficult—even for the engineers who build them—to pinpoint exactly how the AI reached a particular conclusion.
Why AI Seems Like a Black Box:
- Complexity of Models: AI models can involve millions of parameters and intricate mathematical functions, making it difficult to trace the logic behind individual decisions.
- Lack of Explainability: Neural networks and machine learning models don’t inherently explain their decision-making process in the way a human would. This lack of a clear explanation can make AI seem impenetrable.
- Opaque Outputs: AI often delivers investment signals without much context. For instance, it might signal to go long on a stock, but it doesn’t naturally provide a narrative to justify that decision unless specific explainability features are built in.
The Gut Feeling of Human Fund Managers
On the other hand, human fund managers rely on their experience, knowledge, and intuition—sometimes referred to as “gut feeling”—to make investment decisions. While humans can explain their reasoning, their decisions are often influenced by cognitive biases and emotional factors that they may not even be aware of.
How Human Decision-Making Works:
- Experience and Heuristics: Human fund managers draw on their personal experience and learned heuristics (rules of thumb) to make decisions. However, these heuristics can be influenced by past successes and failures, potentially leading to overconfidence or risk aversion.
- Cognitive Biases: Humans are subject to biases, such as confirmation bias (favoring information that confirms pre-existing beliefs) or recency bias (placing too much emphasis on recent events). These biases can distort decision-making and lead to inconsistent outcomes.
- Gut Feeling: In many cases, fund managers rely on intuition when hard data doesn’t point to a clear decision. This gut feeling is shaped by their emotions, past experiences, and subjective interpretation of market signals.
Human Decision-Making: Data Input, Processing, and the Unexplained
Research on human decision-making has shown that the quality of inputs (data) greatly impacts the outcome. However, even when provided with the same data, different individuals often arrive at different conclusions. This is partly because humans process information not just logically but also emotionally. For example, a fund manager might use the same market data as an AI system but interpret it based on personal expectations, stress levels, or market sentiment.
Research Findings on Human Decisions:
- Rationality vs. Emotion: While humans strive for rationality, their decision-making is often influenced by emotions. Studies show that emotional states can significantly impact investment choices, such as risk tolerance or confidence in a particular trade.
- Unconscious Processing: Many of the factors that drive human decisions are processed unconsciously. For instance, the “gut feeling” is not fully explainable and often arises from past experiences that may not be immediately relevant to the current situation.
- Unexplainable Factors: Even experienced fund managers cannot fully explain every decision they make, as their choices may be influenced by complex and often unconscious factors.
AI vs. Human Decision-Making: Which Is More Transparent?
While AI is often seen as a black box, the human decision-making process isn’t fully transparent either. Fund managers may explain their reasoning, but biases, emotions, and intuition often play a role in shaping their decisions—factors that are rarely fully articulated.
Key Differences Between AI and Human Transparency:
- Data-Driven vs. Intuition-Driven: AI is purely data-driven. It analyzes vast amounts of historical and real-time data and identifies patterns that would be impossible for a human to spot. Human fund managers, while using data, also rely heavily on their instincts and subjective interpretations, which are not always reliable or explainable.
- Consistency vs. Variability: AI models, once trained, apply consistent logic across all scenarios. Human decision-making, on the other hand, is inherently variable, affected by mood, stress, and other external factors. An AI might always follow the same rule set, but a fund manager’s gut feeling can change from day to day.
- Emotionless Decisions vs. Emotional Influences: One of AI’s key strengths is its lack of emotional bias. Human decision-making, however, is prone to emotional influences like fear during a market downturn or greed during a bull market. While AI might be seen as cold and mechanical, it’s also free from the emotional pitfalls that affect human judgment.
- Decision by Committee: In human-driven fund management, investment decisions are often made by committees. This process can dilute individual responsibility, as multiple members contribute to the final decision. Factors such as personal sympathies, internal hierarchies, and group dynamics can influence the outcome, making it unclear why a particular investment was approved or rejected. This collective decision-making adds layers of complexity and reduces transparency, as pinpointing the specific reasons behind a decision becomes challenging.
AI at Omphalos: Combining Transparency and Performance
At Omphalos, our AI models are not left entirely as a black box. The key to maintaining transparency is understanding how the model fits into a broader, fully explainable strategy. While the specific predictions made by the AI might not always be explainable due to the complexity of the neural networks, the investment strategies we deploy based on those predictions are clear, transparent, and fully controlled by humans.
How It Works:
- AI-Driven Signals: The AI analyzes vast datasets and generates investment signals based on patterns it detects. These signals are the output of complex models trained on financial data, news, and other relevant inputs.
- Systematic Execution: The execution of trades based on AI signals is done through systematic strategies, which are fully transparent. These strategies define how trades are entered and exited based on the signals provided by AI, ensuring that the actual trade decisions are not left to chance or intuition.
- Human Oversight: While the AI drives the signal generation, human experts are responsible for ensuring that the data fed into the system is of the highest quality. Humans also oversee the consistency of the AI model, stepping in to review the system’s behavior when it performs either better or worse than expected. This oversight ensures that the AI behaves as it should, without introducing risks from unexpected biases or errors.
Systematic Investing: The Role of Transparency
While AI is responsible for analyzing data and generating signals, the actual trade execution process is entirely explainable. At Omphalos, we use systematic investing strategies, which follow clear, rule-based processes for executing trades. This means that every trade can be traced back to a specific signal and rule, providing full transparency for investors.
Systematic Investing Features:
- Clear Rules: Every trade follows a predefined set of rules. There’s no room for emotional decision-making, ensuring that the process is objective and reliable.
- Full Transparency: Unlike the decision-making process of human fund managers, which can be influenced by gut feelings or biases, systematic investing provides full transparency. Investors can clearly see the steps that led to each trade.
- Data-Driven and Consistent: By relying on data rather than human intuition, systematic investing ensures that trades are made consistently, free from the emotional highs and lows that can cloud human judgment.
Conclusion: Is AI Really a “Black Box”?
While AI models may seem like a black box due to their complexity, the strategies that govern them can be fully transparent. By combining the power of AI-driven signals with systematic, rule-based execution, firms like Omphalos can deliver transparent, explainable investment outcomes. Human fund managers, on the other hand, often rely on emotions and gut feelings—factors that are not always fully explainable or consistent.
Ultimately, AI-led investing provides a level of transparency that goes beyond the subjective nature of human decision-making. With the right safeguards in place and a focus on quality data and systematic execution, AI can offer both high performance and transparency, dispelling the myth of the “black box.”
We will continue to explore the differences between AI decision-making and human “gut feeling,” the safeguards in place to prevent AI bias and overfitting, and how AI can become more transparent in the future. The goal is to demystify AI in asset management and show that the “black box” perception is more myth than reality.
Next week, in our final chapter of this series, we will explore how advancements in AI transparency and explainability are helping to bridge the gap between complex AI models and human understanding, ensuring that AI-led investing can be both powerful and fully accountable.
Thank you for following us. We will try to continue to address relevant topics around AI in Asset Management.
If you missed our former editions of “Behind The Cloud”, please check out our BLOG.
© The Omphalos AI Research Team – November 2024
If you would like to use our content please contact press@omphalosfund.com