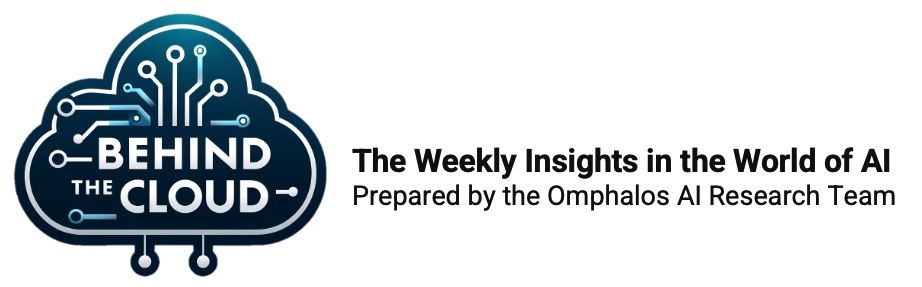
#1 - Behind The Cloud: Time Series Forecasting (1/7)
May 2024
In the dynamic world of finance, where a mere ripple can escalate into a global economic wave, the skill to predict future events holds unprecedented value. We introduce Time Series Forecasting (TSF), a refined analytical method that equips analysts, investors, and policymakers to peer into the financial future with clarity and foresight. This guide launches an seven-week exploration into the depth and breadth of TSF, specially curated for the sharp eyes of institutional investors and journalists.
At its core, TSF is the science (and art) of using historical data to predict future events. This technique is not just confined to the financial sector; its applications span weather forecasting, energy consumption, sales analysis, and beyond. However, in the volatile arena of financial markets, its significance is unparalleled. Despite apparent market unpredictabilities, TSF uncovers stable patterns that, observed over time, can be used to predict future market movements, asset prices, and economic indicators. It hinges on the recognition that while markets may seem chaotic, there is stability in certain aspects that can be reliably forecasted if observed closely.
Why is this crucial for institutional investors and financial journalists? The stakes of making informed decisions are high—where mere milliseconds can translate into millions. Having a predictive advantage could mean the difference between seizing opportunities or missing them completely. For journalists, a deep understanding of TSF provides a tool to interpret market trends and economic projections, enriching their narratives with insightful, forward-looking perspectives. And: It’s important to understand the new born product category of AI-managed funds.
How predictable is the future?
TSF is founded on the idea that historical data contains secrets of the future, waiting to be unlocked. This data is meticulously analyzed to reveal inherent patterns—be they seasonal variances, enduring trends, or cyclical behaviors. Yet, TSF should not be mistaken for a crystal ball; it demands intense analytical effort, sophisticated modeling, and a critical understanding that these forecasts are probabilities, not certainties.
As we dive deeper into the realm of TSF, we will explore a variety of forecasting models, ranging from classic statistical methods to advanced machine learning techniques. Each model offers particular strengths and is suited for specific scenarios, from projecting next hour’s or day’s stock prices to estimating future interest rates.
However, the true essence of TSF transcends mere prediction; it’s about shaping strategic decision-making. For investors, it involves using forecasts to inform portfolio management, mitigate risks, and pinpoint new opportunities. For journalists, it lays the groundwork for stories that not only describe current market conditions but also predict upcoming trends.
Over the next 7 weeks, we will dissect the workings of TSF, its application in finance, and its implementation in real-world scenarios. We will tackle the inherent limitations and challenges of forecasting and consider the future of financial predictions in a world increasingly guided by data.
Join us on this enlightening journey through the intricacies of time series forecasting, where we simplify the complex art of predicting the future, one data point at a time. For both institutional investors and financial journalists, grasping TSF isn’t merely about keeping up with the present—it’s about staying a step ahead of what’s to come.
© The Omphalos AI Research Team – May 2024
If you would like to use our content please contact press@omphalosfund.com
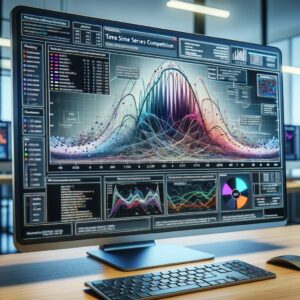