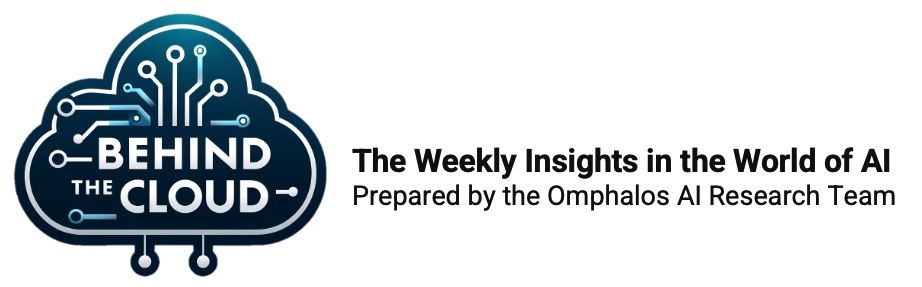
#49 - Behind The Cloud: AI-Powered Insights - Mastering Data-Driven Decision-Making in Finance (7/9)
Data Integrity and Security – Building Robust Systems
May 2025
AI-Powered Insights: Mastering Data-Driven Decision-Making in Finance
Data is no longer just fuel for decision-making—it’s a strategic asset in its own right. In an industry defined by complexity, speed, and uncertainty, mastering the full potential of data is becoming the defining edge in asset management.
In this actual Behind The Cloud series, we explore how Artificial Intelligence is transforming the way financial institutions collect, process, and apply data to make smarter, faster, and more transparent investment decisions. We look beyond the hype, uncovering the architectures, tools, and strategies that turn raw information into meaningful insight.
Data Integrity and Security – Building Robust Systems
In the AI era, data is capital—but without integrity and security, that capital is fragile. Asset managers working with sensitive financial information, proprietary datasets, and complex AI pipelines face growing pressure to ensure that their data is not only accurate, but also secure, traceable, and resilient.
This chapter explores how financial institutions can safeguard the integrity and security of their data, why this matters for model performance and regulatory compliance, and how Omphalos Fund approaches this critical challenge.
What Do We Mean by Data Integrity and Security?
Data integrity refers to the accuracy, consistency, and trustworthiness of data across its lifecycle. Security, on the other hand, involves the protection of that data from unauthorized access, corruption, or loss.
In AI-powered asset management, these two pillars are foundational—not just for compliance, but for decision quality. If data pipelines are corrupted, compromised, or outdated, even the most advanced models will generate flawed outputs.
Why It Matters: The Hidden Risks of Compromised Data
Modern financial systems depend on multi-source, real-time, and often proprietary data. Any failure in integrity or security introduces both operational and reputational risks, including:
- Investment Losses: Flawed or manipulated data can directly lead to poor model decisions and financial underperformance.
- Corrupted Models: Training AI models on biased, incomplete, or manipulated data leads to unreliable predictions.
- Regulatory Violations: Mishandling data, especially under GDPR, MiFID II, or the AI Act, can result in legal penalties.
- Cyber Threats: Ransomware and data breaches can expose sensitive IP and client information.
- Loss of Trust: Stakeholders—clients, regulators, and partners—must trust that data is correct, current, and protected.
Key Principles for Ensuring Data Integrity and Security
- Data Lineage and Provenance
Know where your data comes from, how it has been transformed, and what assumptions have been made. This supports:
- Model explainability
- Regulatory reporting
- Error tracing
- Validation and Cleansing Pipelines
Use automated pipelines to check for anomalies, outliers, or missing values before data is fed into your AI models.
- Access Control and Encryption
Protect sensitive data at rest and in transit using encryption, and implement role-based access to prevent insider threats.
- Version Control for Datasets
Just like code, data should be versioned. This allows for reproducibility and backtesting while ensuring traceability.
- Monitoring and Drift Detection
Continuously monitor data quality metrics and flag shifts in distribution that could affect model performance.
- Incident Response and Recovery Plans
Prepare for the worst. Cybersecurity and data integrity are as much about response as they are about prevention.
Challenges in Real-World Implementation
Even firms with strong infrastructure face real challenges:
- Legacy Systems: Many firms still depend on fragmented or outdated tech stacks that weren’t built for today’s data demands.
- Data Silos: Different teams or vendors manage separate datasets without unified governance.
- Lack of Standards: The industry still lacks clear, consistent benchmarks for AI-specific data governance.
- Balancing Access with Control: Too much restriction can hinder research and model development. Too little opens the door to misuse.
The answer lies in intelligent design, not just tighter controls.
Omphalos Fund: Engineering Trustworthy Data Infrastructure
At Omphalos Fund, data integrity and security are embedded into our AI architecture from day one. We believe that robust data pipelines are not a backend concern—they are a strategic asset.
Our Approach:
- Structured Data Pipelines: We implement automated cleaning, versioning, and validation stages before any data reaches our forecasting models.
- Drift Monitoring: Our systems continuously scan for data anomalies and inconsistencies that could compromise model accuracy.
- Incident Preparedness: We maintain scenario-based recovery plans and test them regularly through simulation exercises.
This approach not only protects our proprietary systems—it also ensures that every model decision can be traced back to clean, reliable inputs.
Conclusion: Integrity and Security as a Competitive Edge
As the complexity and volume of financial data continue to grow, data integrity and security are no longer back-office concerns—they are front-line differentiators. Firms that treat their data like a critical asset will unlock the full potential of AI. Those who don’t will be left managing risk—not returns.
At Omphalos Fund, we see secure, high-integrity data as the foundation of performance, trust, and long-term resilience.
Next week in Behind The Cloud, we’ll explore “Visualization for Decision-Making – Turning Data into Insights”, examining how visual tools help human decision-makers understand and act on the signals AI uncovers.
Stay tuned!
If you missed our former editions of “Behind The Cloud”, please check out our BLOG.
© The Omphalos AI Research Team – May 2025
If you would like to use our content please contact press@omphalosfund.com