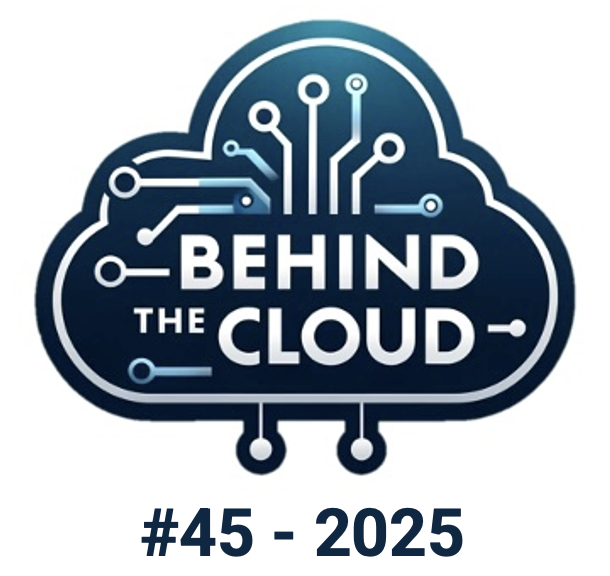
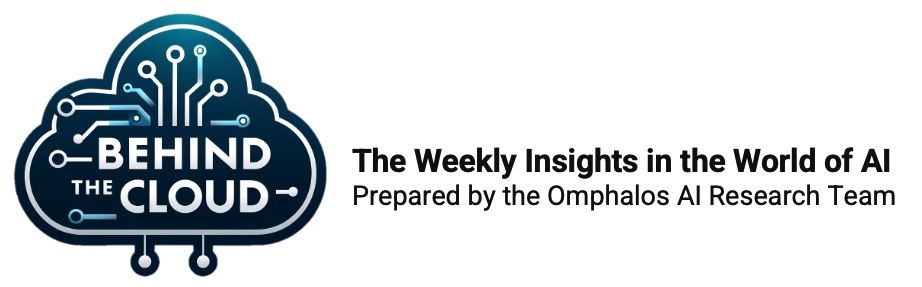
#45 - Behind The Cloud: AI-Powered Insights - Mastering Data-Driven Decision-Making in Finance (3/9)
Integrating Real-Time Market Data into Decision-Making – Staying Ahead of the Curve
April 2025
AI-Powered Insights: Mastering Data-Driven Decision-Making in Finance
Data is no longer just fuel for decision-making—it’s a strategic asset in its own right. In an industry defined by complexity, speed, and uncertainty, mastering the full potential of data is becoming the defining edge in asset management.
In this actual Behind The Cloud series, we explore how Artificial Intelligence is transforming the way financial institutions collect, process, and apply data to make smarter, faster, and more transparent investment decisions. We look beyond the hype, uncovering the architectures, tools, and strategies that turn raw information into meaningful insight.
Integrating Real-Time Market Data into Decision-Making – Staying Ahead of the Curve
In a world where milliseconds matter, the value of near real-time data cannot be overstated. Financial markets are dynamic ecosystems—constantly influenced by macroeconomic events, news flows, market sentiment, and microstructural signals (sometimes including Trump tweets 😄). To succeed in this environment, asset managers must go beyond collecting fast-moving data—they must know how to use it effectively. The real edge lies not in embedding data into models, but in using it to train, inform, and guide model-driven decisions with precision and intent. That means connecting data across systems, curating it with discipline, and applying it where and when it truly matters.
Artificial Intelligence (AI) plays a pivotal role in enabling this shift. While traditional IT systems often process data faster, they lack the capacity to learn, adapt, and generalize from experience. AI-powered systems may not always be the fastest in pure computation, but their real strength lies in their ability to learn from data, recognize complex patterns, and continuously improve decision-making over time. This makes them uniquely suited for transforming near real-time information into strategic action in dynamic market environments.
This chapter examines how real-time data is meaningfully integrated into decision-making workflows—and why doing so strategically is now essential to modern asset management.
Why (Near) Real-Time Matters More Than Ever
In traditional workflows, analysts and portfolio managers review daily or weekly data snapshots to inform decisions. But in fast-moving markets, delayed insights can translate into missed opportunities – or unmanaged risks.
What makes real-time data powerful is not just speed, but the ability to turn velocity into value through intelligent application:
- Market Responsiveness: Immediate reactions to economic releases, earnings surprises, or breaking news.
- Volatility Management: Faster recalibration of positions in response to shifting market conditions.
- Signal Precision: Improved forecasting accuracy by integrating the most current market behavior into model inputs.
- Short-Term Alpha: Real-time trading signals offer competitive edge in arbitrage, execution strategies, and intraday positioning.
Real-time capabilities are no longer a luxury – they are the new baseline for decision-relevant data usage in AI-powered asset management.
How AI Ingests and Uses Real-Time Data
The true power of real-time data lies in how it is operationalized. AI doesn’t just collect this data – it interprets it, learns from it, and acts on it dynamically.
Core Components of Real-Time Data Integration:
- Streaming Data Pipelines: Real-time feeds from exchanges, news APIs, macroeconomic calendars, and alternative sources are continuously piped into AI systems.
- Low-Latency Infrastructure: Fast computational environments ensure that insights are processed and deployed with minimal delay.
- Event-Based Triggers: AI agents react to changing market conditions – price thresholds, volatility spikes, sentiment shifts – within seconds.
- Dynamic Model Updates: Some models adjust their parameters or risk tolerances in real time, learning from the latest inputs.
It’s not just about receiving data – it’s about integrating it into workflows that can act reflexively and intelligently.
Applications in Asset Management
Real-time market data is reshaping how decisions are made, monitored, and adjusted across the investment lifecycle.
Key applications include:
- Execution Algorithms: AI-driven systems dynamically optimize trade execution by analyzing order book depth, volume imbalances, and liquidity pockets in real time.
- Nowcasting Models: Real-time economic indicators—like credit card transactions or port activity—feed short-term macro predictions.
- Sentiment-Based Allocation: Rapidly processed insights from social media, analyst commentary, or news feeds can influence short-term portfolio adjustments or hedging strategies—often with a short delay, but still significantly faster than traditional methods.
- Risk Triggers: Real-time systems flag emerging risks—such as geopolitical events, commodity shocks, or currency devaluations—before they ripple through portfolios.
Challenges of Using Real-Time Data
Turning high-frequency data into high-quality decisions presents a unique set of challenges:
- Noise vs. Signal: Markets are full of short-term fluctuations. Separating actionable insights from noise requires model calibration and contextual understanding.
- Infrastructure Demands: Building and maintaining low-latency environments—often involving specialized on-premise setups or edge computing solutions, rather than standard cloud infrastructure—can be resource-intensive and complex.
- Cost of Data Feeds: Real-time sources are often premium-priced and must be evaluated for strategic relevance and ROI.
- Data Drift and Model Stability: AI systems must remain robust and consistent even as input data evolves rapidly in high-volatility regimes.
Omphalos Fund: Built for the Real-Time Age
At Omphalos Fund, we don’t just receive real-time data – we integrate it meaningfully and act on it with discipline and precision. For us, time isn’t just a metric – it’s a strategic input to our investment logic.
Our Approach:
- Event-Sensitive AI Agents: Our systems are built with AI agents that adapt strategies based on news, order flow, and intraday sentiment signals.
- Volatility-Aware Models: We incorporate semi real-time volatility indicators to fine-tune position sizing and execution timing.
- Connected Infrastructure: Our modular systems connect real-time inputs directly to forecasting, rebalancing, and hedging engines.
- Human Oversight: Our team validates AI outputs in real time, ensuring strategic alignment even under market pressure.
This architecture ensures that we don’t just observe markets in motion – we act within them intelligently.
Conclusion: The Shift from Static to Streaming Intelligence
Real-time market data isn’t just faster – it’s fundamentally different. It represents the flow of market consciousness, capturing behavior, emotion, and structural shifts as they unfold. But to benefit from it, firms must do more than collect data – they must understand how to use it in ways that are predictive, adaptive, and risk-aware.
At Omphalos Fund, we’ve built our platform not just to observe the market—but to move with it. By embedding real-time thinking into our models, we’re making decisions that reflect the present moment with clarity and conviction.
Next week in “Behind The Cloud” we’ll explore “Beyond Text – Leveraging Unstructured Data in AI Models” – examining how audio, video, images, and non-tabular information are becoming essential components of the next-generation financial data stack.
Stay tuned.
If you missed our former editions of “Behind The Cloud”, please check out our BLOG.
© The Omphalos AI Research Team – April 2025
If you would like to use our content please contact press@omphalosfund.com