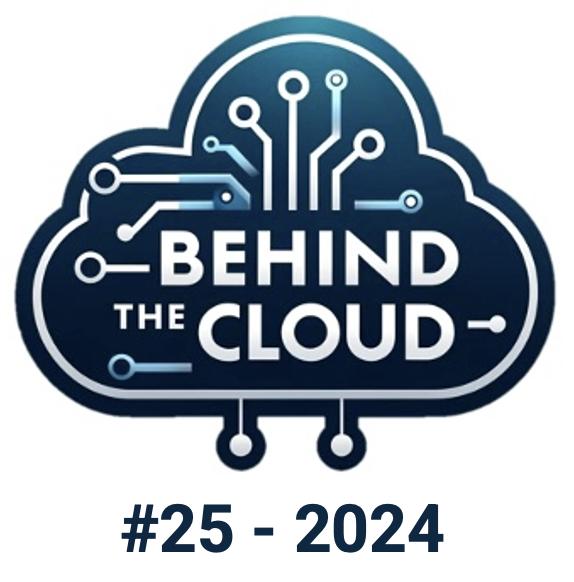
The Future of AI Transparency: Moving Beyond the ‘Black Box’
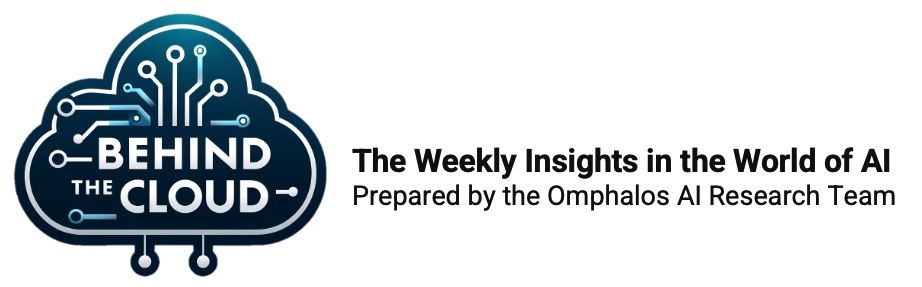
#25 - Behind The Cloud: Demystifying AI in Asset Management: Is It Really a Black Box? (6/6)
The Future of AI Transparency: Moving Beyond the 'Black Box'
November 2024
As AI continues to evolve and integrate further into asset management, the need for transparency becomes increasingly vital. The perception of AI as a “black box” can create hesitancy among investors and asset managers alike. To build trust and optimize the value AI offers, it’s essential to look at how transparency can be enhanced and what the future holds for AI in the financial industry. This chapter will explore the advancements in explainability tools, industry practices, and how these developments are moving AI beyond the “black box” perception.
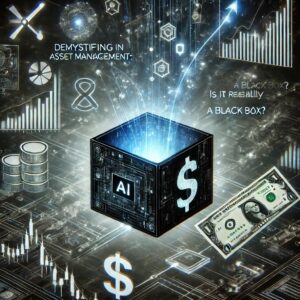
The Importance of Transparency in AI
Transparency in AI is more than just a buzzword; it’s a necessity for responsible and effective AI deployment in asset management. Transparency allows stakeholders to understand the logic behind investment decisions, ensuring that AI-driven strategies align with the firm’s values, risk appetite, and compliance requirements.
Why Transparency Matters
- Building Trust: Investors and clients are more likely to trust AI-led strategies if they understand how decisions are made.
- Regulatory Compliance: Financial regulations often require clear documentation of decision-making processes. Transparent AI helps firms meet these standards.
- Enhanced Accountability: Transparency facilitates oversight, making it easier to identify and rectify issues before they impact performance or trust.
New Tools for Explainability
Recent advancements in AI research have focused on creating tools and methods that improve the interpretability of complex models. While traditional machine learning models like decision trees and linear regression are inherently interpretable, more advanced models like deep neural networks require specialized tools for explainability.
Key Developments in Explainability
- Explainable AI (XAI): This field focuses on developing algorithms and methods that make AI decision-making processes more interpretable. XAI tools can highlight which features had the most impact on a given decision, providing a clearer picture of how the model reached its conclusion.
- Model-Agnostic Tools: Techniques like LIME (Local Interpretable Model-agnostic Explanations) and SHAP (SHapley Additive exPlanations) can be applied to any model type, offering explanations that help users understand why a specific prediction was made.
- Visualization Dashboards: Advanced visualization tools now allow asset managers to see how different variables affect AI predictions, creating a more interactive way to explore AI behavior and decision-making.
Industry Practices Promoting Transparency
The financial industry is increasingly adopting practices that enhance transparency in AI systems. These practices are helping to set new standards for how AI is integrated and explained within investment processes.
Best Practices for Transparency
- Regular Audits: Conducting regular reviews of AI models ensures they are functioning as intended and helps catch potential biases or overfitting early.
- Documentation and Reporting: Detailed documentation of AI algorithms, their training data, and decision-making processes is crucial for compliance and internal reviews.
- Integration of Human Oversight: Ensuring that human experts are involved in the monitoring and validation of AI outputs adds a layer of accountability and trust.
- Awareness of Adversarial Attacks: Adversarial attacks are a growing risk in AI, especially in high-stakes areas like finance. These attacks involve subtle manipulations in input data, intentionally crafted to mislead the AI model. Asset managers need to implement robust safeguards, such as adversarial training, to help AI models recognize and resist such manipulations. By staying vigilant to this risk, firms can better protect their models and the integrity of AI-driven decisions.
Moving Beyond the ‘Black Box’
The idea of moving beyond the “black box” is not just about making AI understandable but ensuring that it complements human expertise rather than replacing it. By combining AI’s analytical power with human judgment, asset management firms can create a transparent, efficient, and effective investment process.
How to Move Forward
- Adopt Hybrid Models: Integrating explainable AI with human-led oversight allows firms to leverage the strengths of both while ensuring transparency.
- Invest in Training: Equip teams with the skills to understand and interpret AI outputs. This training ensures that the people managing AI systems can explain and justify its decisions.
- Carefully Select Training Data: The quality and relevance of training data directly impact AI model performance and acceptance. By carefully curating data, firms can better explain models and avoid biases or irrelevant patterns that could obscure transparency. Selection processes should focus on representative data to ensure fair, reliable model predictions.
- Promote Open Communication: Maintain clear lines of communication with investors and stakeholders about how AI is used, its benefits, and the safeguards in place to prevent errors or biases.
Omphalos Fund’s Vision for Transparent AI
At Omphalos Fund, transparency is a core principle in how we approach AI-led investing. We recognize that while AI provides unparalleled analytical capabilities, it must operate in a way that aligns with the expectations of our clients and regulatory standards.
Our Commitment
- Explainable Models: We use state-of-the-art explainability tools to provide clarity on how our AI models generate investment signals.
- Human Oversight: Our team of experts regularly monitors and validates AI outputs to ensure they are consistent with our strategies and risk parameters.
- Continuous Improvement: We remain dedicated to exploring new technologies and methodologies that enhance the transparency and reliability of our AI systems.
Conclusion: A Transparent Future for AI in Asset Management
The future of AI in asset management lies in achieving a balance between advanced analytics and transparency. With ongoing developments in explainability tools, best practices, and a commitment to open communication, the “black box” perception of AI can be overcome. At Omphalos Fund, we aim to set the benchmark for transparent, data-driven investing that clients can trust.
This concludes our final chapter in the series “Demystifying AI in Asset Management: Is It Really a Black Box?”
We hope it’s provided valuable insights into the evolving role of AI, transparency, and innovation in finance.
As we move forward, we’re already exploring new themes for our next series, and we’d love to hear your thoughts! If there are topics you’re curious about or areas you’d like us to dive deeper into, please feel free to share your ideas with us. Stay tuned for more insights as we continue our journey in Behind the Cloud.
If you missed our former editions of “Behind The Cloud”, please check out our BLOG.
© The Omphalos AI Research Team – November 2024
If you would like to use our content please contact press@omphalosfund.com