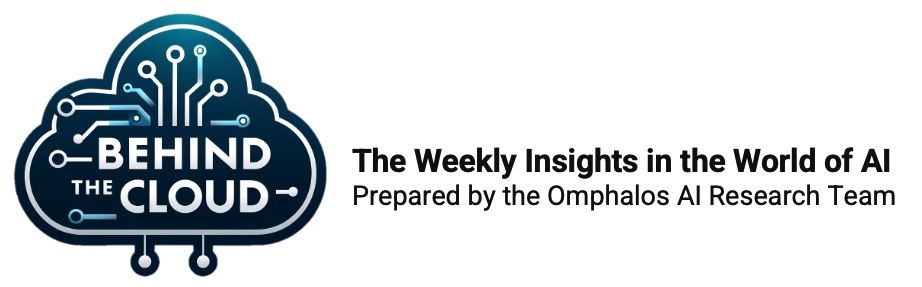
#20 - Behind The Cloud: Demystifying AI in Asset Management: Is It Really a Black Box? (1/6)
The Black Box Myth – What Makes AI Seem Incomprehensible?
October 2024
Artificial Intelligence (AI) has been a game changer in asset management, providing data-driven insights and strategies that were once impossible for human analysts. But as AI becomes more prevalent, one concern keeps surfacing:
Is AI really a “black box”?
Many believe that AI operates in ways that are mysterious, hard to explain, and ultimately uncontrollable. This chapter will explore the roots of this myth and clarify what makes AI seem so opaque.
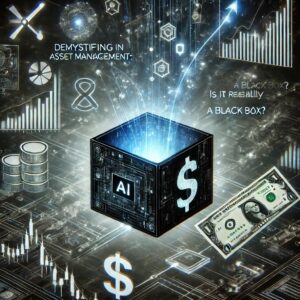
Understanding the “Black Box” Concern
The term “black box” in AI refers to a system where we know the inputs (data) and the outputs (decisions), but the process in between seems hidden or overly complex. In asset management, this can lead to a lack of trust. Investors might wonder how AI arrives at decisions that affect their portfolios. The perception is that AI-driven systems make decisions based on invisible calculations, which humans can’t follow or fully understand.
This concern largely arises from the complexity of certain AI models, especially deep learning models. These models use multiple layers of algorithms to process large amounts of data, but the intricate workings of these layers are not easily understood, even by data scientists. This lack of clarity can lead to the “black box” label, particularly in industries where transparency is essential.
Why Some AI Models Seem Like a “Black Box”
Not all AI models are created equal. Some techniques, like Support Vector Machines (SVM) or decision trees, are relatively easier to interpret. They show clear relationships between inputs and outputs. However, more advanced models, like neural networks (including deep learning systems with multiple layers), are much more complex. These models learn patterns from vast amounts of data but don’t provide simple explanations for their decisions.
The “black box” problem is most prominent in these complex systems. Deep learning, for example, uses many layers of artificial neurons to process data. Each layer refines the data further until a decision is made, but explaining how each neuron arrived at its conclusion is difficult. For asset managers, this can create a challenge. They need to trust the AI to make investment decisions, but they also need to understand how it arrived at those decisions.
The Importance of Data Quality
One key factor that impacts AI’s decision-making is the quality of the data being fed into the model. Unlike humans, who can adjust their decisions in real-time based on intuition or new information, AI is only as good as the data it receives. High-quality input data is crucial for AI to make accurate and reliable predictions. Humans control this critical aspect by choosing the right set of data from reliable sources. By ensuring data quality, humans can guide AI systems to operate with greater accuracy, reducing the “black box” effect.
If the data is flawed – whether it’s biased, incomplete, or irrelevant – the AI model will produce equally flawed results, regardless of how advanced the algorithm is. This is why careful selection, cleansing, and management of input data are essential tasks that humans must oversee. Data scientists and asset managers need to ensure that the inputs fed into AI systems are relevant, reliable, and reflective of the current market environment.
Common Misconceptions About AI Transparency
One common misconception is that all AI is a black box. This is not true. Many models, especially in machine learning, are interpretable. For example, in simpler algorithms like decision trees or Support Vector Machines (SVM), it’s easier to track how decisions are made based on the data fed into the system.
Another misconception is that AI systems are left to run without oversight. In reality, asset managers constantly monitor AI models, tweaking them as needed and ensuring they perform within expected parameters. Even in complex systems, experts can often analyze the factors driving decisions, such as market trends or stock performance data, even if the exact algorithmic path isn’t fully understood.
Finally, some believe that AI models make decisions in isolation from human input. This is also incorrect. In asset management, AI usually works alongside human experts. These experts provide context, review AI-generated insights, and apply their own knowledge to finalize decisions.
Balancing Complexity and Transparency
The complexity of AI models can’t be denied. However, complexity doesn’t necessarily mean lack of control. In asset management, it’s critical to find a balance between using sophisticated AI systems and maintaining a level of transparency. The goal is not to oversimplify AI but to ensure that the models are understandable enough for stakeholders to trust them.
Many firms are working on tools and methods to improve AI transparency. These efforts include building more interpretable models or creating interfaces that show decision paths in simpler terms. Some advanced AI platforms now offer tools to visualize decision-making processes, making it easier for asset managers to explain how decisions were made to their clients.
Not All AI is a Black Box
One important point to highlight is that not all AI is a black box. Many models, such as decision trees or ensemble methods, offer clear pathways from data input to decision output. These models can explain how specific factors, like market volatility or earnings reports, contribute to the final decision. In fact, many asset managers prefer to use these more transparent models in specific scenarios where clear reasoning is needed.
On the other hand, deep learning models, which are highly effective in recognizing patterns in huge datasets, may seem less transparent but offer unmatched precision in prediction. This trade-off between interpretability and performance is a key discussion in asset management, especially when using AI to make high-stakes investment decisions.
Why Does the Black Box Myth Persist?
So, why does the idea of AI being a “black box” continue to persist? There are a few reasons:
- Complexity of the Models: As mentioned, the most advanced AI models, like neural networks with complex architectures and multiple layers, are inherently complex. This makes it difficult for non-experts to understand how they work, which can lead to a perception of opacity.
- Lack of Explainability Tools: Until recently, there were limited tools available to explain AI decisions. The industry is now catching up with new methods to interpret decisions made by even the most complex models, but it’s still early days.
- Fear of Losing Control: For asset managers used to traditional methods, handing over decision-making to a machine feels risky. The less they can explain about how the machine makes decisions, the more they feel they’ve lost control of the process.
- Mistrust of New Technologies: Any new technology, especially one as impactful as AI, comes with its own set of fears and misunderstandings. For many, the term “black box” is just a convenient way to describe a technology they don’t fully understand yet.
Conclusion: Setting the Stage for Transparency
AI in asset management doesn’t have to be a black box. While some models may seem complex and hard to interpret, the majority of AI systems in use today are transparent and explainable. As the technology continues to evolve, we can expect more tools and methods to make even the most complex models more understandable.
In the coming weeks, we will explore the differences between AI decision-making and human “gut feeling,” the safeguards in place to prevent AI bias and overfitting, and how AI can become more transparent in the future. The goal is to demystify AI in asset management and show that the “black box” perception is more myth than reality.
Stay tuned for Week 2, where we’ll dive into the decision-making process of both humans and AI, revealing how the two compare in transparency and reliability.
Thank you for following us. We will try to continue to address relevant topics around AI in Asset Management.
If you missed our former editions of “Behind The Cloud”, please check out our BLOG.
© The Omphalos AI Research Team – October 2024
If you would like to use our content please contact press@omphalosfund.com