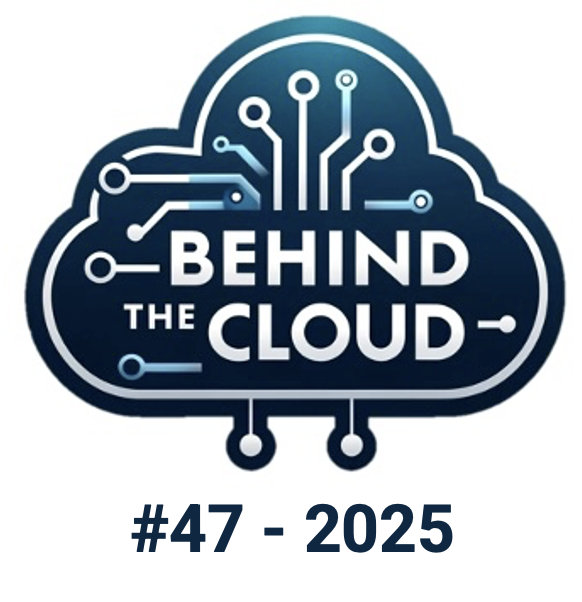
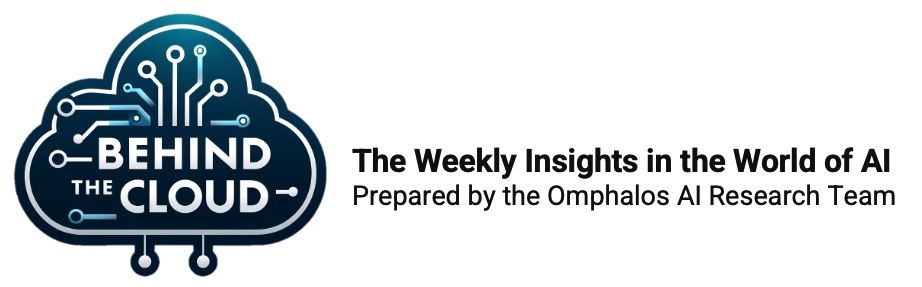
#47 - Behind The Cloud: AI-Powered Insights - Mastering Data-Driven Decision-Making in Finance (5/9)
Building Proprietary Data Sets – A Competitive Edge in Asset Management
May 2025
AI-Powered Insights: Mastering Data-Driven Decision-Making in Finance
Data is no longer just fuel for decision-making—it’s a strategic asset in its own right. In an industry defined by complexity, speed, and uncertainty, mastering the full potential of data is becoming the defining edge in asset management.
In this actual Behind The Cloud series, we explore how Artificial Intelligence is transforming the way financial institutions collect, process, and apply data to make smarter, faster, and more transparent investment decisions. We look beyond the hype, uncovering the architectures, tools, and strategies that turn raw information into meaningful insight.
Building Proprietary Data Sets – A Competitive Edge in Asset Management
In an industry where many signals are already priced in and widely accessible, the most valuable data is often the data you own. Proprietary datasets—unique, internally generated, or exclusive data sources—are fast becoming one of the strongest differentiators in AI-driven asset management. But simply owning unique datasets isn’t enough. Their true value is realized only when they’re actively used—linked with other data sources, structured effectively, and integrated into models that can turn them into insight.
In this chapter, we explore why proprietary data gives investors a sustainable edge, how it’s built and maintained, and what role it plays in developing AI strategies that outperform in a crowded landscape.
Why Proprietary Data Matters More Than Ever
Most traditional datasets used in investing—price feeds, macroeconomic indicators, earnings estimates—are public and commoditized. While still important, they rarely offer a true advantage on their own.
Proprietary data, by contrast, provides:
- Exclusive Insights: Patterns and features that competitors cannot replicate.
- Signal Differentiation: Unique alpha sources for machine learning models.
- Strategic Flexibility: Control over how data is structured, cleaned, and used.
- Historical Signal Performance: A direct view into how proprietary signals and strategies have behaved over time, enabling more precise model training, validation, and confidence in forward-looking applications.
In the age of AI, where data is the fuel, having proprietary pipelines can be more important than having access to the best model.
Types of Proprietary Data in Asset Management
There is no one-size-fits-all. Proprietary data can take many forms depending on strategy, focus, and operational capacity.
Examples include:
- Custom Market Indicators: Internal measures of liquidity, sentiment, or volatility based on granular order-book data.
- Research-Enhanced Signals: Analyst reports or ESG scoring methodologies developed in-house.
- Client Interaction Data: Aggregated behavioral insights from investor platforms or advisory interfaces (with full data privacy compliance).
- Operational Data: Internal trading logs, execution quality stats, or risk analytics outputs used for pattern mining.
- Proprietary Forecasting Features: Time-series transformations or feature-engineering outputs developed uniquely for machine learning models.
- Real Transaction and Signal Data: Proprietary logs of actual investment signals and executed transactions—offering unparalleled value over synthetic or backtested alternatives due to their authenticity, behavioral context, and real-world response capture.
Each dataset becomes a foundation for signals that are less likely to be crowded and more adaptable to evolving market conditions. Increasingly, firms also treat internal unstructured sources—like investor calls, research notes, or client feedback—as proprietary datasets. With the help of LLMs, these can be transformed into structured indicators, such as sentiment time series or topic trends.
How AI Leverages Proprietary Data
AI models thrive on volume, variety, and relevance. Proprietary datasets offer all three—especially when tailored to the modeling process.
Key Benefits of Proprietary Data in AI Workflows:
- Improved Signal-to-Noise Ratio: Higher relevance often translates into better model performance.
- Better Generalization: Models trained on unique data avoid overfitting to public datasets already used by competitors.
- More Effective Feature Engineering: Greater control enables deeper transformations and more meaningful model inputs.
- Discovery of Unique Market Patterns: Proprietary datasets can reveal previously unseen relationships or emerging signals that are invisible to models trained on commoditized data.
Well-maintained proprietary data also becomes a strategic asset across multiple investment models, from alpha forecasting to risk modeling and ESG scoring.
The true strength of proprietary data emerges when it’s combined with external or alternative data sources. Connecting internal data with broader market signals enables asset managers to uncover hidden relationships, refine forecasts, and enhance model resilience.
Challenges in Building Proprietary Data Sets
Building a valuable proprietary data pipeline is not easy—and that’s what makes it valuable. It requires commitment, infrastructure, and cross-functional collaboration.
Common Challenges Include:
- Data Collection Infrastructure: Establishing clean, consistent, and real-time data feeds from internal or external sources.
- Data Quality Control: Ensuring completeness, consistency, and accuracy of data over time.
- Storage and Access: Managing cost-effective, scalable, and secure infrastructure for data retention and retrieval.
- Integration with Models: Bridging the gap between raw data and model-ready features.
- Governance and Process Discipline: Enforcing strict procedures for how data is collected, transformed, and used—ensuring that each dataset is as useful and reliable as possible throughout the modeling pipeline.
Above all, the data must be relevant. Building just for the sake of ownership doesn’t help unless the data improves decision-making.
Omphalos Fund: Data That Others Don’t Have
At Omphalos Fund, proprietary data isn’t just a resource—it’s a strategy. We’ve invested heavily in building datasets that capture the nuances of market dynamics and support our AI-first approach to forecasting and portfolio management. We also explore how to transform unstructured internal data into structured signals using LLMs—for example, extracting time-series indicators from text-based client records, reports, or communications.
Our Approach:
- Custom Feature Libraries: We generate proprietary time-series features designed specifically for our machine learning and forecasting engines.
- Internal Risk and Signal Logs: We collect and structure AI-driven decision outputs to refine and test future model behavior.
- Structured Labeling: Through consistent backtesting, we generate outcome-based labels to support supervised model training with high-quality ground truth.
- Quality Assurance Processes: We maintain rigorous standards for how proprietary data is processed, versioned, and integrated into production systems.
This commitment enables us to build signals others can’t replicate—giving us a competitive edge in both performance and adaptability.
Conclusion: Own the Data, Own the Edge
In the race to apply AI in finance, those who control the data pipelines will shape the investment landscape. Proprietary datasets aren’t just about secrecy or exclusivity—they’re about control, quality, and long-term edge.
At Omphalos Fund, we treat proprietary data as a core asset class in itself—just as critical as capital or talent. It’s not about having more data. It’s about having the right data, structured the right way, to power the next generation of investment decisions.
Next week in Behind The Cloud, we explore “AI and Alternative Data – Opportunities and Challenges”, turning our focus to non-traditional data sources—from satellite imagery to geolocation—and how they’re changing the rules of asset management.
Stay tuned!
If you missed our former editions of “Behind The Cloud”, please check out our BLOG.
© The Omphalos AI Research Team – May 2025
If you would like to use our content please contact press@omphalosfund.com