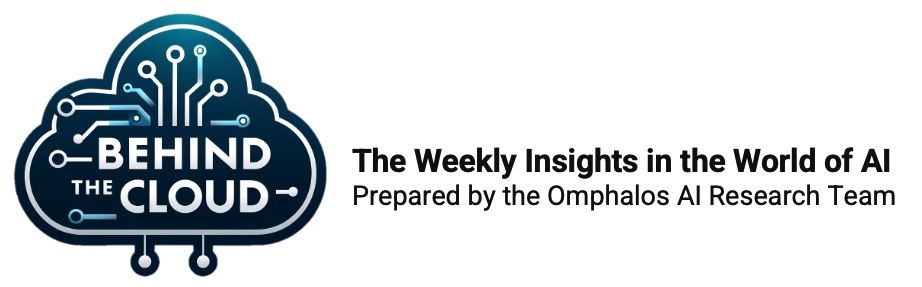
#46 - Behind The Cloud: AI-Powered Insights - Mastering Data-Driven Decision-Making in Finance (4/9)
Beyond Text – Leveraging Unstructured Data in AI Models
May 2025
AI-Powered Insights: Mastering Data-Driven Decision-Making in Finance
Data is no longer just fuel for decision-making—it’s a strategic asset in its own right. In an industry defined by complexity, speed, and uncertainty, mastering the full potential of data is becoming the defining edge in asset management.
In this actual Behind The Cloud series, we explore how Artificial Intelligence is transforming the way financial institutions collect, process, and apply data to make smarter, faster, and more transparent investment decisions. We look beyond the hype, uncovering the architectures, tools, and strategies that turn raw information into meaningful insight.
Beyond Text – Leveraging Unstructured Data in AI Models
Most traditional financial models have been built on structured data — order books, time-series prices, economic indicators, and balance sheets. But in today’s world, some of the most valuable insights live outside of neatly formatted spreadsheets.
Think of quarterly earnings calls, satellite imagery of retail parking lots, CEO interviews, investor chatrooms, or even handwritten notes on scanned documents. These are examples of unstructured data — and they’re rapidly becoming essential inputs for next-generation AI models.
In this chapter, we explore how unstructured data is reshaping decision-making in asset management, what types of data offer the greatest edge, and how AI can process them effectively to unlock insights that others miss.
The Rise of Unstructured Data in Finance
According to IDC, more than 80% of the world’s data is unstructured—and that percentage is growing. For asset managers, this presents both a challenge and an opportunity: how to extract meaningful information from vast, messy, and often unlabeled data sources.
Key Types of Unstructured Data Relevant to Asset Management
- Text: News articles, social media, analyst reports, earnings call transcripts
- Audio: CEO tone and delivery in earnings calls, investor podcasts, regulatory hearings
- Visuals: Satellite imagery, corporate logos, sentiment from facial expressions in interviews
- Documents: PDFs, scanned contracts, non-standard regulatory filings
These data types provide context, tone, nuance, and signals that structured data alone cannot capture.
How AI Extracts Insights from Unstructured Data
Unstructured data is, by definition, messy. AI—especially through Natural Language Processing (NLP), computer vision, and speech recognition—has made it possible to convert these chaotic inputs into structured insights.
Core AI Techniques
- Natural Language Processing (NLP): Tools like transformers and LLMs analyze sentiment, extract entities, summarize documents, and detect bias or uncertainty in written and spoken text.
- Speech-to-Text + Voice Analysis: Audio from earnings calls or interviews is transcribed and analyzed for pacing, tone, hesitancy, and emphasis.
- Computer Vision: Satellite images or on-the-ground photos are processed to count vehicles, track store activity, or assess supply chain flows.
- Multi-Modal Models: New-generation AI systems can combine inputs from multiple data formats—such as pairing text with satellite images—to enhance decision quality.
Applications in Asset Management
Unstructured data is increasingly being used to gain a competitive edge—especially in areas where traditional signals are already crowded.
Key Applications Include
- Sentiment Forecasting: Analyzing executive tone and language during earnings calls to anticipate post-earnings drift.
- Event Detection: Identifying early signals of geopolitical instability from regional news and social media platforms.
- Activity Monitoring: Using satellite imagery or mobile location data to track economic activity at retail chains, ports, or construction sites.
- Thematic Investing: Extracting forward-looking themes from investor letters, regulatory documents, or industry interviews.
Challenges of Using Unstructured Data
Despite its promise, working with unstructured data is resource-intensive and often requires dedicated infrastructure and specialized expertise.
Key Challenges
- Labeling and Annotation: Unstructured data often needs to be manually tagged or labeled to train supervised learning models effectively.
- High Noise Levels: Social media, for example, contains a mix of signal and noise that must be filtered carefully to avoid spurious conclusions.
- Interpretability: NLP and computer vision models can be difficult to audit or explain—raising regulatory and internal governance concerns.
- Storage and Scalability: Audio, image, and large text corpora require scalable storage and retrieval systems to remain useful in real-time settings.
Omphalos Fund: Structuring the Unstructured
At Omphalos Fund, we believe that the best insights come from where others aren’t yet looking. We’ve embedded the ability to process unstructured data into the DNA of our AI platform—transforming raw information into differentiated signals.
Our Approach
While many specifics remain proprietary, we can share that we are actively exploring the use of large language models (LLMs) to extract structured, decision-ready values from unstructured inputs. This includes converting free text, transcribed speech, and other qualitative information into quantifiable indicators such as time series data. By doing so, we aim to enrich our forecasting models with layers of insight that structured data alone cannot provide.
Sorry to say so, but this touches our IP in its heart. We can not disclose more. We hope you will understand.
Conclusion: Looking Beyond Spreadsheets
The future of data-driven finance lies beyond the structured world of rows and columns. As more value hides in the unstructured—and as AI becomes more capable of reading, listening, and seeing like a human—those who can unlock this data will gain a real edge.
At Omphalos Fund, we believe that structuring the unstructured is the next frontier of alpha generation. It’s not just about having more data—it’s about making more of the data we have.
Next week in Behind The Cloud, we’ll explore “Building Proprietary Data Sets – A Competitive Edge in Asset Management”, highlighting how firms are gaining long-term strategic advantage by investing in data collection and ownership.
Stay tuned.
If you missed our former editions of “Behind The Cloud”, please check out our BLOG.
© The Omphalos AI Research Team – May 2025
If you would like to use our content please contact press@omphalosfund.com