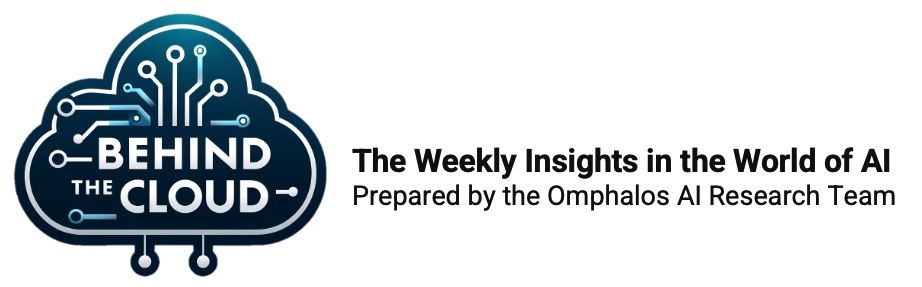
#32 - Behind The Cloud: Beyond the Frontier - What’s Next for AI Systems in Asset Management? (7/8)
The Next Steps for AI Transparency – Building Trust While Embracing Complexity
January 2025
As Artificial Intelligence (AI) continues to evolve and reshape the financial landscape, transparency is becoming a cornerstone for its responsible deployment. In asset management, trust is paramount, and ensuring accountability in AI-driven systems fosters confidence among investors and stakeholders. Transparency is not only a priority in itself but also an essential part of the broader ethical discussion surrounding the use of AI.
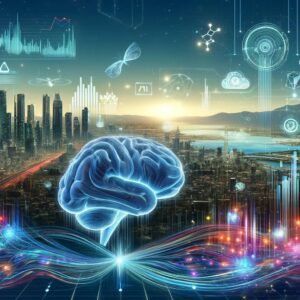
This chapter explores the next steps in advancing AI transparency, outlining the challenges, best practices, and opportunities to create systems that are powerful, trustworthy, and as interpretable as possible.
Why Transparency Matters in AI
Transparency in AI refers to the ability to explain and understand how decisions are made by AI systems. In asset management, transparency is critical for several reasons:
Key Drivers:
- Investor Confidence: Transparent systems provide clarity on how investment decisions are made, building trust with clients and stakeholders.
- Regulatory Compliance: Financial regulations increasingly focus on AI accountability. Transparent systems help firms adhere to these standards.
- Alignment with Values: Transparency ensures that AI aligns with a firm’s objectives and values, reducing reputational risks and fostering sustainable growth.
While transparency is central to the ethical use of AI, it’s important to recognize that complete explainability, particularly in deep learning systems, may not always be possible due to the inherent complexity of neural networks. This limitation must be acknowledged and addressed through other measures to build trust.
Challenges in Achieving Transparency
Despite its importance, transparency in AI systems faces significant challenges:
Major Challenges:
- Complexity of Neural Networks: Advanced AI models, particularly deep neural networks, involve intricate computations that can be difficult to interpret. This “black box” nature makes full transparency challenging.
- Bias in Data and Algorithms: Datasets can introduce unintended biases that affect fairness and accuracy, making transparency even more critical to identify and address these issues.
- Accountability in Automated Decisions: When AI systems make decisions, determining responsibility for outcomes remains a gray area.
- Balancing Performance and Transparency: Simplifying models to enhance interpretability can sometimes reduce their effectiveness. The challenge lies in achieving a balance that doesn’t compromise results.
Acknowledging these challenges while striving for as much clarity as possible is key to advancing trust in AI systems.
Best Practices for Transparency and Ethical Alignment
To promote trust and accountability, asset managers can adopt best practices that enhance transparency and support ethical use.
Enhancing Transparency
- Explainable AI (XAI): Tools like SHAP (SHapley Additive exPlanations) and LIME (Local Interpretable Model-Agnostic Explanations) clarify how AI models arrive at decisions, providing valuable insights to stakeholders.
- Documentation: Maintaining detailed records of model design, training processes, and data sources ensures a clear audit trail.
- Interactive Interfaces: Visualization dashboards allow stakeholders to explore how variables influence AI predictions, fostering greater understanding.
Proactive Regulation and Compliance
- Alignment with Standards: Staying updated with frameworks such as the EU’s AI Act ensures compliance and accountability in AI systems.
- Risk Mitigation Frameworks: Implementing AI-specific risk strategies addresses ethical concerns and minimizes unintended outcomes.
By embedding these practices, asset managers can build systems that are not only powerful but also aligned with ethical expectations.
The Role of Transparency in Asset Management
In asset management, where decisions carry significant financial implications, transparency plays a pivotal role in delivering value to stakeholders.
Real-World Applications:
- Risk Assessment: Transparent AI models help investors understand the risks associated with specific strategies, promoting informed decision-making.
- ESG Integration: Transparency ensures AI aligns with Environmental, Social, and Governance (ESG) goals, supporting fairness and inclusivity in investment processes.
- Client Trust: Demonstrating transparency in AI-driven strategies fosters long-term client relationships and enhances brand reputation.
At Omphalos Fund, transparency is a guiding principle in how we develop and deploy AI systems, ensuring they remain aligned with our commitment to delivering value and trust to our clients.
Innovations Driving Transparency in AI
The future of transparency in AI will be shaped by advancements in technology, governance, and stakeholder expectations. Key innovations include:
Emerging Trends:
- Federated Learning: This approach trains models on decentralized data sources, enhancing privacy and reducing potential biases while maintaining transparency.
- Explainable Neural Networks: Efforts to design more interpretable neural network architectures are paving the way for greater transparency in complex systems.
- Real-Time Auditing: Advanced tools are enabling continuous monitoring of AI systems, detecting and rectifying issues in real-time.
By adopting these innovations, asset managers can ensure their AI systems remain transparent, reliable, and aligned with industry standards.
Omphalos Fund’s Commitment to Transparency
At Omphalos Fund, transparency is at the heart of our AI-driven solutions. While complete explainability may not always be feasible due to the complexity of neural networks, we are committed to ensuring that our systems remain as interpretable and accountable as possible.
Our Approach:
- Explainability Tools: Leveraging advanced tools like XAI, we make AI decisions as transparent as possible for clients and stakeholders.
- Accountability Measures: Regular audits and governance frameworks ensure our AI systems operate within defined ethical boundaries.
- Client Engagement: We prioritize open communication, ensuring that clients understand how AI supports our investment strategies and the safeguards in place.
Conclusion: A Transparent AI Future
Transparency is central to building trust in AI systems, especially in asset management, where decisions have far-reaching implications. While complete transparency may not always be achievable, acknowledging these limitations and striving for maximum clarity is essential for fostering confidence and accountability.
At Omphalos Fund, we view transparency as a critical part of the ethical conversation around AI, embedding it into every layer of our systems. By staying at the forefront of AI innovation, we aim to deliver solutions that inspire trust, enhance performance, and set the standard for responsible AI in asset management.
This concludes our seventh chapter in the series “𝗕𝗲𝘆𝗼𝗻𝗱 𝘁𝗵𝗲 𝗙𝗿𝗼𝗻𝘁𝗶𝗲𝗿: 𝗪𝗵𝗮𝘁’𝘀 𝗡𝗲𝘅𝘁 𝗳𝗼𝗿 𝗔𝗜 𝗦𝘆𝘀𝘁𝗲𝗺𝘀 𝗶𝗻 𝗔𝘀𝘀𝗲𝘁 𝗠𝗮𝗻𝗮𝗴𝗲𝗺𝗲𝗻𝘁?”
We hope it’s provided valuable insights into the cutting-edge developments shaping the next era of Artificial Intelligence (AI).
Next week, in our final chapter of this series, “The Vision Ahead – Redefining the Asset Management Landscape”, we synthesize all topics to outline a future vision for AI in asset management. Discover how advancements in neural networks, quantum computing, RAG pipelines, and governance frameworks will converge to create AI systems that are transparent, reliable, and indispensable. Stay tuned!
If you missed our former editions of “Behind The Cloud”, please check out our BLOG.
© The Omphalos AI Research Team – January 2025
If you would like to use our content please contact press@omphalosfund.com