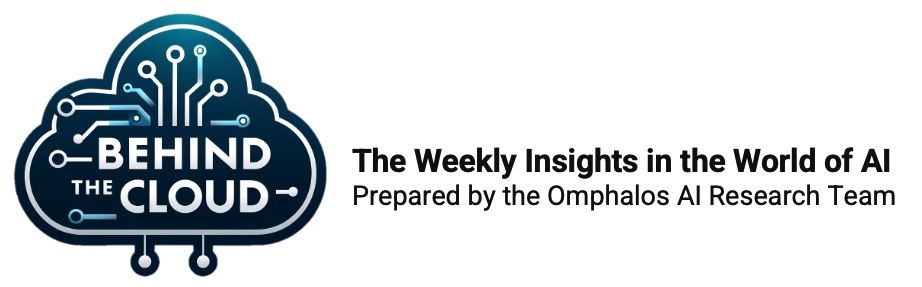
#30 - Behind The Cloud: Beyond the Frontier - What’s Next for AI Systems in Asset Management? (5/8)
The Future of Machine Learning and Deep Learning – Beyond Today’s Neural Networks
December 2024
Machine learning (ML) and deep learning (DL) have been the driving forces behind some of the most transformative technologies in recent years, from natural language processing to predictive analytics in finance. However, as these techniques reach their current limits, researchers and practitioners are exploring the next frontier of ML and DL, paving the way for even greater advancements.
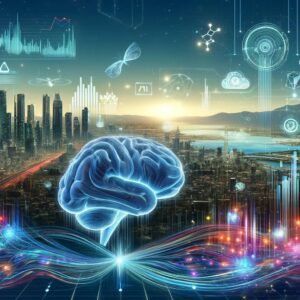
In this chapter, we’ll delve into the future of ML and DL, examining the innovations beyond today’s neural networks and their implications for asset management.
The Evolution of Machine Learning and Deep Learning
Machine learning (ML) encompasses a wide range of algorithms, including linear regression, support vector machines, decision trees, and neural networks. Neural networks, especially shallow ones, are a subset of ML. Deep learning (DL), on the other hand, refers specifically to neural networks with multiple layers, allowing them to learn complex patterns in large datasets.
Key Achievements of ML and DL
- Image and Speech Recognition: Deep neural networks have achieved near-human accuracy in recognizing objects and understanding spoken language.
- Natural Language Processing (NLP): Transformer-based models, such as GPT and BERT, have revolutionized how machines understand and generate text.
- Predictive Analytics: ML algorithms, including both traditional and deep learning approaches, are widely used in asset management to predict market trends, optimize portfolios, and assess risks.
Despite these achievements, ML and DL face challenges, including high computational demands, difficulties in explainability, and the risk of overfitting. The future of ML and DL lies in addressing these limitations while unlocking new possibilities.
Emerging Trends in Machine Learning and Deep Learning
Several groundbreaking trends are shaping the future of ML and DL, promising to redefine their capabilities and applications.
1. Beyond Neural Networks: Neuromorphic Computing
Inspired by the human brain, neuromorphic computing involves designing hardware and algorithms that mimic neural and synaptic behavior. Unlike traditional neural networks, these systems process information in parallel and are energy-efficient.
Implications for Asset Management:
- Faster data processing for real-time market analysis.
- Enhanced decision-making systems that integrate unstructured data sources like news sentiment and social media trends.
2. Federated Learning: Privacy-Preserving Collaboration
Federated learning enables multiple parties to train a shared ML model without exchanging raw data, addressing privacy concerns. Each participant trains the model locally and shares updates rather than sensitive data.
Implications for Asset Management:
- Collaborative risk modeling among financial institutions without violating data privacy regulations.
- Enhanced AI models trained on diverse datasets while maintaining client confidentiality.
3. Self-Supervised Learning (SSL): Reducing Data Dependency
Self-supervised learning leverages unlabeled data to train models, significantly reducing the reliance on expensive and time-consuming data labeling processes.
Implications for Asset Management:
- Faster development of AI models that incorporate diverse market data.
- Improved efficiency in training predictive models for asset pricing and portfolio optimization.
4. Explainable AI (XAI): Bridging Transparency Gaps
As ML and DL models become more complex, explainable AI focuses on improving their interpretability. Techniques such as Local Interpretable Model-Agnostic Explanations (LIME) and SHapley Additive exPlanations (SHAP) are gaining traction.
Implications for Asset Management:
- Greater transparency in algorithmic trading decisions, enhancing investor confidence.
- Easier compliance with regulatory requirements for explainability in financial decision-making.
5. Transformers Beyond LLMs: Generalized Architectures
While Transformers are best known for their impact on Large Language Models (LLMs), their architecture is increasingly being adapted for other domains, such as image recognition, time-series analysis, and even reinforcement learning. The versatility of Transformers lies in their ability to process and relate data across different modalities, opening new frontiers in AI applications.
Implications for Asset Management:
- Improved Pattern Recognition: Enhanced ability to detect and analyze trends in financial time-series data, aiding in predictive analytics.
- Multi-Modal Insights: Integration of textual, numerical, and even visual data to provide a more comprehensive view of market dynamics and portfolio performance.
- Cross-Domain Applications: Leveraging Transformer-based models for risk assessment, sentiment analysis, and real-time decision-making across diverse financial instruments.
Challenges in the Next Phase of ML and DL
Despite their potential, the future of ML and DL comes with several challenges:
- Computational Demands: Advanced models require significant computational resources, making scalability a concern for smaller firms.
- Data Quality and Bias: Ensuring high-quality, unbiased datasets remains critical for producing reliable predictions.
- Interpretability vs. Complexity: Striking a balance between model accuracy and transparency is an ongoing challenge, especially in highly regulated industries like finance.
- Ethical Considerations: As ML and DL become more autonomous, addressing ethical concerns such as algorithmic fairness and data privacy is crucial.
The Future of ML and DL in Asset Management
For asset managers, the advancements in ML and DL offer opportunities to enhance decision-making, improve efficiency, and deliver greater value to clients.
What Lies Ahead:
- Next-Generation Predictive Models: ML and DL techniques will enable more accurate forecasting of market trends and economic indicators.
- Personalized Investment Strategies: By analyzing individual client preferences and behavior, ML models can create tailored portfolios that align with specific goals.
- Advanced Risk Management: DL’s pattern recognition capabilities will help firms detect early warning signals of market disruptions, ensuring proactive risk mitigation.
Omphalos Fund: Pioneering Advanced Machine Learning in Asset Management
At Omphalos Fund, we are dedicated to harnessing the most advanced developments in ML and DL to enhance our investment strategies. By aligning state-of-the-art algorithms with our expertise in asset management, we continue to push the boundaries of AI-driven investing.
Our Initiatives:
- Research and Development: Focusing on developing more advanced types of neural networks, particularly optimized for time series forecasting, to address the dynamic and complex nature of financial markets.
- Transparency Focus: Incorporating explainable AI tools to ensure clarity and accountability in our decision-making processes, reinforcing trust with clients and stakeholders.
- Tailored Algorithms: Adapting general ML and DL models to the specific needs of investment strategies, ensuring that our approaches are fine-tuned for optimal performance in market forecasting, risk management, and portfolio optimization.
Conclusion: The Next Era of ML and DL
The future of machine learning and deep learning is set to go beyond today’s neural networks, unlocking transformative possibilities for innovation and impact. By combining these advancements with breakthroughs in quantum computing, cloud technology, and data-driven systems, the potential for AI in asset management expands exponentially. Together, these technologies will redefine how data is analyzed, decisions are made, and strategies are executed in a hyper-connected financial world.
At Omphalos Fund, we see this convergence as a unique opportunity to drive transparency, efficiency, and innovation. The integration of quantum computing for solving complex optimization problems, the scalability of cloud infrastructure, and the precision of next-generation ML and DL models enable us to deliver unparalleled value to our clients. By staying ahead of these cutting-edge developments, we are shaping the foundation for smarter, more adaptive, and sustainable investing strategies.
This concludes our fifth chapter in the series “𝗕𝗲𝘆𝗼𝗻𝗱 𝘁𝗵𝗲 𝗙𝗿𝗼𝗻𝘁𝗶𝗲𝗿: 𝗪𝗵𝗮𝘁’𝘀 𝗡𝗲𝘅𝘁 𝗳𝗼𝗿 𝗔𝗜 𝗦𝘆𝘀𝘁𝗲𝗺𝘀 𝗶𝗻 𝗔𝘀𝘀𝗲𝘁 𝗠𝗮𝗻𝗮𝗴𝗲𝗺𝗲𝗻𝘁?”
We hope it’s provided valuable insights into the cutting-edge developments shaping the next era of Artificial Intelligence (AI).
The next chapter of “Behind The Cloud” will be published after the Christmas break in January. We’ll explore “Fine-Tuning and Morphing Large Language Models – Tailoring Intelligence to Specific Needs” uncovering how customization can unlock new possibilities for AI systems in asset management. Stay tuned!
If you missed our former editions of “Behind The Cloud”, please check out our BLOG.
© The Omphalos AI Research Team – December 2024
If you would like to use our content please contact press@omphalosfund.com