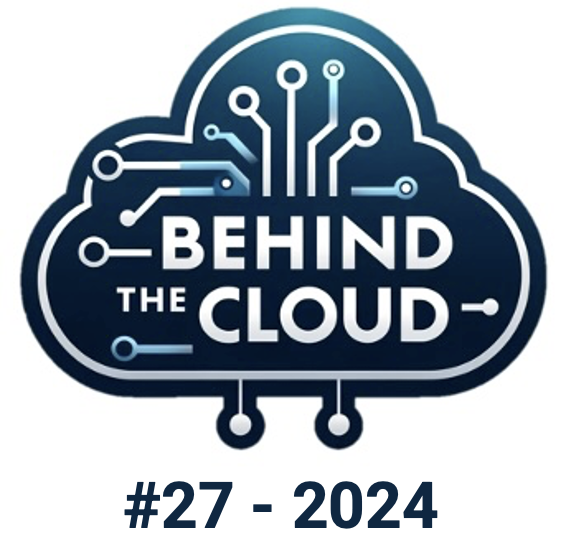
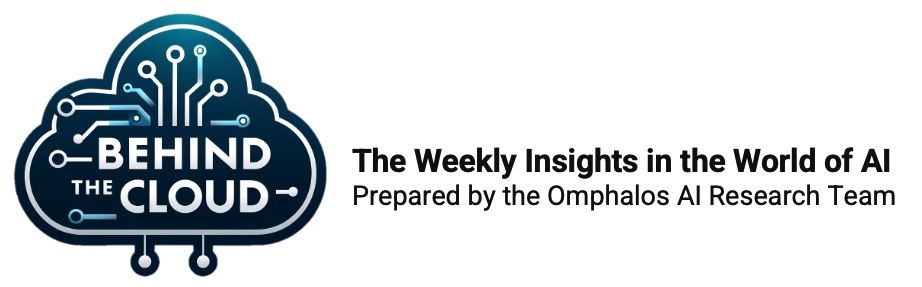
#27 - Behind The Cloud: Beyond the Frontier - What’s Next for AI Systems in Asset Management? (2/8)
Advanced Multi-Agent Systems – Coordinating Intelligence for Smarter Outcomes
November 2024
Artificial Intelligence (AI) is no longer confined to standalone systems solving individual problems. The future lies in collaboration, where multiple AI agents work together to achieve complex objectives. Known as multi-agent systems, these networks of intelligent entities communicate, coordinate, and even compete to deliver smarter and more dynamic outcomes. In this chapter, we explore how multi-agent systems are advancing AI capabilities and their transformative potential in asset management.
What Are Multi-Agent Systems?
Multi-agent systems consist of multiple AI agents, each with specialized roles or tasks, working together to achieve a shared objective. Each agent brings a unique focus, such as analyzing data, drawing conclusions, critiquing ideas, planning work, or executing specific functions. These agents can communicate, share insights, and adjust their behaviors in response to changes in their environment or the actions of other agents. The result is a system that is greater than the sum of its parts—more flexible, adaptive, and capable of handling dynamic, real-world challenges.
In asset management, multi-agent systems can:
- Optimize portfolio strategies by simulating various market conditions.
- Detect and mitigate risks in real time through distributed monitoring.
- Enhance decision-making by pooling insights from different data streams and analyses.
For example, one agent might focus on analyzing macroeconomic indicators, another on real-time market data, and a third on client-specific objectives. Together, these agents provide a comprehensive and coordinated investment strategy.
How Multi-Agent Systems Work
Multi-agent systems operate on principles of cooperation, competition, and autonomy.
Here’s how they function:
- Autonomy: Each agent operates independently, making decisions based on its specific goals and data inputs.
- Coordination: Agents communicate and collaborate, ensuring their actions align with the system’s overarching objective.
- Adaptability: Agents learn from their interactions and adapt to changes, whether in the market, the data, or the behavior of other agents.
In the context of asset management, a multi-agent system might involve agents specializing in areas like equities, bonds, commodities, and alternative investments. These agents exchange information to create a balanced and optimized portfolio.
Applications in Asset Management
The use of multi-agent systems in asset management is still emerging, but their potential is enormous.
Key applications include:
- Portfolio Optimization: Multi-agent systems can evaluate different asset classes simultaneously, identifying opportunities and balancing risks across diverse markets. Agents specializing in equities can share insights with those focused on fixed income, enabling a holistic portfolio strategy.
- Market Simulation and Forecasting: Agents can simulate market behaviors under various scenarios, such as interest rate changes or geopolitical events. This helps firms stress-test their strategies and anticipate market reactions.
- Fraud Detection and Compliance: By distributing monitoring tasks among specialized agents, multi-agent systems can detect anomalies, flag suspicious transactions, and ensure compliance with regulatory standards in real time.
- Client Personalization: Agents dedicated to understanding individual clients’ preferences can collaborate with investment-focused agents to deliver tailored strategies and communication.
Challenges in Multi-Agent Systems
While the benefits are clear, multi-agent systems present unique challenges:
- Coordination Complexity: Ensuring that agents work cohesively without conflicting actions can be difficult, especially in high-stakes environments like trading.
- Scalability: As the number of agents increases, so does the complexity of their interactions. Asset managers must ensure that systems remain efficient and responsive as they scale.
- Decision Accountability: When multiple agents contribute to a decision, tracing the rationale behind the final action can be challenging, which is a concern for compliance and transparency.
- Training Processes: Developing a robust training process for multiple agents to ensure they can learn both independently and collaboratively poses a significant challenge. This includes aligning their objectives and optimizing their shared learning outcomes.
- Convergence: Achieving convergence in multi-agent systems—where all agents align their actions and reach a mutually acceptable solution—can be particularly complex, especially in dynamic or competitive environments.
Advancements Driving Multi-Agent Systems
Recent technological advancements are making multi-agent systems more practical and impactful:
- Reinforcement Learning (RL): Agents learn optimal strategies through trial and error, improving their performance over time.
- Hierarchical Systems: By organizing agents into hierarchies, systems can better manage complex tasks. For example, a high-level agent might oversee risk management, while lower-level agents handle specific asset classes.
- Communication Protocols: Improved protocols enable seamless information sharing between agents, ensuring coordination and reducing the risk of conflicts.
- Latest Multi-Agent Models: OpenAI’s GPT-4.0 represents a prime example of a multi-agent system, with specialized components working collaboratively to enhance functionality and deliver sophisticated outputs. This multi-agent structure exemplifies the growing importance of coordinated intelligence in modern AI architectures.
In asset management, these advancements allow multi-agent systems to dynamically adjust to changing market conditions, creating more resilient and adaptable investment strategies.
Omphalos Fund: Embracing Multi-Agent Collaboration
At Omphalos Fund, we are exploring the integration of multi-agent systems into our investment processes. By leveraging these advanced systems, we aim to enhance decision-making, optimize portfolio strategies, and improve risk management.
How We Use Multi-Agent Systems:
- Collaborative Intelligence: Our multi-agent setups allow specialized agents to share insights and strategies, creating a comprehensive view of market opportunities.
- Real-Time Adaptation: Agents respond dynamically to market changes, ensuring that our strategies remain effective even in volatile conditions.
- Dynamic Portfolio Adjustment and Optimization: By leveraging multi-agent coordination, we can adjust and optimize portfolios dynamically in response to shifting market conditions, enabling better alignment with investment goals and risk parameters.
- Human Oversight: While the agents collaborate, human experts oversee the system to ensure it aligns with our goals and risk parameters.
This hybrid approach combines the analytical power of multi-agent systems with human judgment, delivering robust and transparent investment solutions.
Conclusion: Unlocking the Potential of Coordination
Advanced multi-agent systems represent a significant leap forward in AI capabilities. By enabling coordinated intelligence, these systems can tackle complex, multi-dimensional problems that single-agent models struggle with. In asset management, their ability to optimize portfolios, simulate markets, and enhance compliance offers a powerful competitive advantage.
At Omphalos Fund, we are committed to staying at the forefront of these developments, integrating multi-agent systems to create smarter, more adaptive investment strategies.
This concludes our second chapter in the series “𝗕𝗲𝘆𝗼𝗻𝗱 𝘁𝗵𝗲 𝗙𝗿𝗼𝗻𝘁𝗶𝗲𝗿: 𝗪𝗵𝗮𝘁’𝘀 𝗡𝗲𝘅𝘁 𝗳𝗼𝗿 𝗔𝗜 𝗦𝘆𝘀𝘁𝗲𝗺𝘀 𝗶𝗻 𝗔𝘀𝘀𝗲𝘁 𝗠𝗮𝗻𝗮𝗴𝗲𝗺𝗲𝗻𝘁?”
We hope it’s provided valuable insights into the cutting-edge developments shaping the next era of Artificial Intelligence (AI).
Next week in Behind the Cloud, we’ll dive into “Retrieval-Augmented Generation (RAG) Pipelines – Delivering Precision to LLMs”, exploring how this technology ensures AI systems work with the right data for the right decisions. Stay tuned!
If you missed our former editions of “Behind The Cloud”, please check out our BLOG.