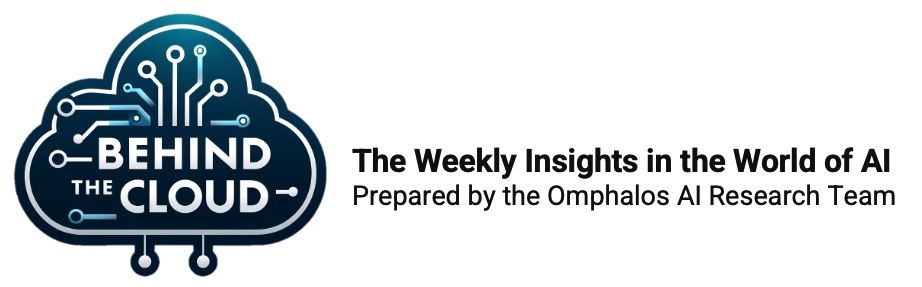
#6 - Behind The Cloud: Time Series Forecasting (6/7)
June 2024
While the allure of predicting financial markets through Time Series Forecasting (TSF) is undeniable, it’s pivotal to approach this endeavor with a clear understanding of its inherent risks and limitations. This week, we navigate through the turbulent waters of uncertainty that surround financial forecasting, shedding light on the challenges and how best to mitigate them.
The Nature of Market Volatility
Financial markets are inherently volatile, influenced by an amalgamation of factors ranging from economic indicators and corporate performance to geopolitical events and regulatory changes. This volatility presents a significant challenge to TSF, as historical data patterns may not always predict future movements accurately, especially in the face of sudden market shifts.
Model Overfitting and Underfitting
A common pitfall in TSF is overfitting, where a model is so finely tuned to past data that it fails to generalize to future data. Conversely, underfitting occurs when a model is too simplistic to capture the underlying patterns in the data. Both scenarios can lead to inaccurate forecasts, underscoring the importance of model validation and regular updates based on new data.
The Black Swan Events
Introduced as a term by Nassim Nicholas Taleb‘s to financial markets, black swan events are unpredictable and carry significant impact. These rare but impactful events pose a considerable challenge to TSF models, which, by their nature, rely on historical data patterns that may not account for the unforeseeable.
Ethical Considerations and Market Impact
The use of TSF and automated trading based on predictive models raises ethical considerations, including the potential for market manipulation and the exacerbation of market volatility. Institutional investors must navigate these ethical waters carefully, ensuring that their forecasting and trading practices contribute to market integrity.
Mitigating Risks
Mitigating the risks associated with TSF involves a multifaceted approach:
- Diversification: Avoid reliance on a single forecasting model or market assumption. Diversifying strategies and asset allocations can reduce the impact of inaccurate forecasts.
- Dynamic Model Updating: Regularly update forecasting models with new data and adjust them in response to changing market conditions to maintain their accuracy and relevance.
- Risk Management: Implement robust risk management strategies, including stop-loss orders and hedging, to protect investments from adverse movements predicted by TSF models or unforeseen events.
In conclusion, while TSF offers a powerful lens through which to view potential future market movements, it comes with its set of challenges and uncertainties. By acknowledging these limitations and adopting strategies to mitigate risks, institutional investors and financial professionals can navigate the complexities of financial forecasting with greater confidence and integrity. As we look to the future, the evolving landscape of financial analysis and forecasting promises new tools and methodologies to tackle these challenges, guided by the lessons learned from navigating the uncertain seas of financial markets.
Stay tuned for our next post, where we will close our first series about TSF. We will look beyond the horizon, including the future outlook for our Omphalos Fund.
If you missed our former edition of “Behind The Cloud”, please check out our BLOG.
© The Omphalos AI Research Team – June 2024
If you would like to use our content please contact press@omphalosfund.com
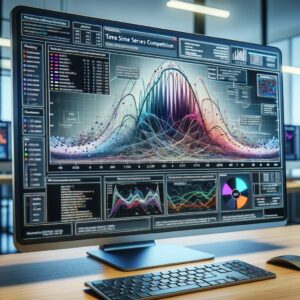